②anaconda + tensorflow2.6.2 + cuda11.2 + cudnn8.1安装使用过程
(建议配合查看官网教程)1.安装 Anaconda2.下载官网的模型Tensorflow model链接1(Tensorflow model)GitHub - tensorflow/models: Models and examples built with TensorFlowhttps://github.com/tensorflow/models解压到C:\tensorflow2并重命名为mo
①测试tensorflow的gpu是否好用
在python解释器中输入如下代码进行测试
import tensorflow as tf
tf.test.is_gpu_available()
如果最后打印出来True,那就说明你成功了。
如果打印出来False,那么可能是你的cuda、cudnn、环境变量、独立显卡、tensorflow 版本等问题引起的,一般不会输出错误信息,这时,你就需要继续寻找教程了,拜拜
如果缺少什么cusolver64_11.dll等 dll文件,百度下载放在指定位置就可以了(如果是 anaconda环境,注意cuda和cuudn版本,conda list查看cudatoolkit 和cudnn版本与 tensorflow版本是否对应)
②anaconda + tensorflow2.6.2 + cuda11.2 + cudnn8.1安装使用过程
(建议配合查看官网教程)
1.安装 Anaconda
2.下载官网的模型Tensorflow model
链接1(Tensorflow model)GitHub - tensorflow/models: Models and examples built with TensorFlow
解压到C:\tensorflow2并重命名为models
3.下载官网的ssd预训练模型
链接2(SSD)GitHub - tensorflow/models: Models and examples built with TensorFlow
下载解压到C:\tensorflow1\models\research\object_detection
4.下载文件库(tensorflow训练的例子)
链接3(文件库)
https://github.com/EdjeElectronics/TensorFlow-Object-Detection-API-Tutorial-Train-Multiple-Objects-Windows-10
下载解压到C:\tensorflow1\models\research\object_detection
5.下载protuf
链接4(protuf)https://github.com/google/protobuf/releases
安装protuf (非常容易出错,我这里选择的是win版 3.4) 将bin文件夹中的【protoc.exe】放到C:\Windows 并cmd进入models\research\ 这个目录下
执行
protoc object_detection/protos/*.proto --python_out=.
三.设置conda虚拟环境
C:\> conda create -n tensorflow2 pip python=3.6.7
C:\> activate tensorflow2
(tensorflow1) C:\> conda install tensorflow-gpu=2.6.2
(tensorflow1) C:\> conda install -c anaconda protobuf
(tensorflow1) C:\> pip install pillow
(tensorflow1) C:\> pip install lxml
(tensorflow1) C:\> pip install Cython
(tensorflow1) C:\> pip install contextlib2
(tensorflow1) C:\> pip install jupyter
(tensorflow1) C:\> pip install matplotlib
(tensorflow1) C:\> pip install pandas
(tensorflow1) C:\> pip install opencv-python
设置python环境变量,在终端执行:
Set PYTHONPATH=C:\tensorflow1\models;C:\tensorflow1\models\research;C:\tensorflow1\models\research\slim
四.编译Protobuf
cd C:\tensorflow1\models\research
protoc object_detection/protos/*.proto --python_out=.
复制C:\tensorflow2\models\research\object_detection\packages\tf2里面的setup.py
到C:\tensorflow1\models\research目录下
执行:
python setup.py build
python setup.py install
(如果python setup.py install执行时下载包太慢或者下载不了,到阿里云镜像官网找对应的包的.whl文件下载后放在C:\anaconda\pkgs目录下)
终端执行pip install C:\anaconda\pkgs\包名 进行安装
之后执行 pip install tf-nightly ,自动下载tensorflow执行环境
五.标注图片
将训练图片和测试图片分别放在
C:\tensorflow1\models\research\object_detection\images\
中的train和test文件夹下
要是使用例子的图片集,注意xml的图片路径要修改(不会图片集标注百度吧)
六.生成训练数据
运行
(tensorflow1) C:\tensorflow1\models\research\object_detection> python xml_to_csv.py
会在 \object_detection\images中生成两个文件
train_labels.csv 和 test_labels.csv
修改object_detection\目录下的generate_tfrecord.py
要修改的部分是
# TO-DO replace this with label map
def class_text_to_int(row_label):
if row_label == 'nine':
return 1
elif row_label == 'ten':
return 2
elif row_label == 'jack':
return 3
elif row_label == 'queen':
return 4
elif row_label == 'king':
return 5
elif row_label == 'ace':
return 6
else:
None
将这部分内容中的row_label==’ ’ 修改为要训练的类别,请根据自己的情况适当增删
比如
# TO-DO replace this with label map
def class_text_to_int(row_label):
if row_label == 'basketball':
return 1
elif row_label == 'shirt':
return 2
elif row_label == 'shoe':
return 3
else:
None
然后生成TFRecord 文件
python generate_tfrecord.py --csv_input=images\train_labels.csv --image_dir=images\train --output_path=train.record
python generate_tfrecord.py --csv_input=images\test_labels.csv --image_dir=images\test --output_path=test.record
会在object_detection\目录下生成train.record和test.record文件
七.创建Label Map
label map 文件为object_detection\training\labelmap.pbtxt
修改其内容,原内容是
item {
id: 1
name: 'nine'
}
item {
id: 2
name: 'ten'
}
item {
id: 3
name: 'jack'
}
item {
id: 4
name: 'queen'
}
item {
id: 5
name: 'king'
}
item {
id: 6
name: 'ace'
}
修改为自己的训练类别和对应ID,ID就是第7步中每个类别返回的数字
比如
if row_label == 'basketball':
return 1
则在labelmap.pbtxt中修改为
item {
id: 1
name: 'basketball'
}
八.配置训练文件
将C:\tensorflow2\models\research\object_detection\configs\tf2里面的
ssd_mobilenet_v2_320x320_coco17_tpu-8.config 文件复制到object_detection\training文件夹中
然后修改该文件
1.num_classes: 标签种类个数
- fine_tune_checkpoint: "C:/tensorflow2/models/research/object_detection/ssd_mobilenet_v2_320x320_coco17_tpu-8/checkpoint/ckpt-0"
- fine_tune_checkpoint_type: "detection"
3.train_input_reader: {
label_map_path: "C:/tensorflow2/models/research/object_detection/trainning/labelmap.pbtxt"
tf_record_input_reader {
input_path: "C:/tensorflow2/models/research/object_detection/train.record"
}
}
4.eval_input_reader: {
label_map_path: "C:/tensorflow2/models/research/object_detection/trainning/labelmap.pbtxt"
shuffle: false
num_epochs: 1
tf_record_input_reader {
input_path: "C:/tensorflow2/models/research/object_detection/test.record"
}
}
batch_size 调整为1-6,太大带不起
- 开始训练
重新训练时需要清除training\中除了labelmap和ssd_mobilenet。。。以外的所有文件,否则会报错
训练执行:
python model_main_tf2.py --logtostderr --model_dir=training/ --pipeline_config_path=training/ssd_mobilenet_v2_320x320_coco17_tpu-8.config
继续训练,将fine_tune_checkpoint:
"C:/tensorflow2/models/research/object_detection/training/ckpt-21"
十.默认设定的迭代次数是20000次,当迭代次数达到最大后,模型训练结束,我们接着进入到training文件夹下可以看到有如下生成的文件:
查看已训练好模型的收敛程度:
D:\Program Files\models\research\object_detection>
tensorboard --logdir training --bind_all
从上图还是可以看出Loss呈下降趋势 ,实际效果如何还需要进一步评估。
十一:导出.pb文件
D:\Program Files\models-master\research\object_detection>
创建文件夹 inference_graph
(1)tf1版本:导出frozen_inference_graph.pb文件。
python export_inference_graph.py --input_type image_tensor --pipeline_config_path training/ssd_mobilenet_v2_320x320_coco17_tpu-8.config --trained_checkpoint_prefix training/ckpt-40 --output_directory inference_graph
(2)tf2版本:导出saved_model.pb,该文件中包含了我们训练好的检测器以及网络架构信息和参数信息等。
python exporter_main_v2.py --input_type image_tensor --pipeline_config_path=training/ssd_mobilenet_v2_320x320_coco17_tpu-8.config --trained_checkpoint_dir=training --output_directory=training/train_export
运行得到的结果如下图所示:
十二.测试Tensorflow模型
写一个Object_detection_image_LLL.py
######## Image Object Detection Using Tensorflow-trained Classifier #########
#
# Author: Evan Juras
# Date: 1/15/18
# Description:
# This program uses a TensorFlow-trained neural network to perform object detection.
# It loads the classifier and uses it to perform object detection on an image.
# It draws boxes, scores, and labels around the objects of interest in the image.
## Some of the code is copied from Google's example at
## https://github.com/tensorflow/models/blob/master/research/object_detection/object_detection_tutorial.ipynb
## and some is copied from Dat Tran's example at
## https://github.com/datitran/object_detector_app/blob/master/object_detection_app.py
## but I changed it to make it more understandable to me.
# Import packages
import os
import cv2
import numpy as np
from PIL import Image
import tkinter
import matplotlib
matplotlib.use('TkAgg')
import matplotlib.pyplot as plt
import time
import tensorflow as tf
from object_detection.utils import label_map_util
from object_detection.utils import config_util
from object_detection.utils import visualization_utils as viz_utils
from object_detection.builders import model_builder
from six import BytesIO
import sys
# This is needed since the notebook is stored in the object_detection folder.
sys.path.append("..")
# Import utilites
from utils import label_map_util
from utils import visualization_utils as vis_util
# 正向传播函数
def get_model_detection_function(model):
"""Get a tf.function for detection."""
@tf.function
def detect_fn(image):
"""Detect objects in image."""
image, shapes = model.preprocess(image)
prediction_dict = model.predict(image, shapes)
detections = model.postprocess(prediction_dict, shapes)
return detections, prediction_dict, tf.reshape(shapes, [-1])
return detect_fn
# Grab path to current working directory
CWD_PATH = os.getcwd()
# Name of the directory containing the object detection module we're using
IMAGE_NAME = 'test3.jpg'
# Path to image
PATH_TO_IMAGE = os.path.join(CWD_PATH,IMAGE_NAME)
# 构建检测模型并加载trained_model权值
pipeline_config = os.path.join(CWD_PATH,'training\\train_export\\','pipeline.config')
model_dir = 'training\\train_export\\checkpoint\\'
# Load pipeline config and build a detection model
# 加载 pipeline config 然后读取检测模型
configs = config_util.get_configs_from_pipeline_file(pipeline_config)
model_config = configs['model']
detection_model = model_builder.build(model_config=model_config, is_training=False)
# 恢复模型
ckpt = tf.compat.v2.train.Checkpoint(model=detection_model)
ckpt.restore(os.path.join(CWD_PATH,model_dir, 'ckpt-0')).expect_partial()
# 加载模型权重
detect_fn = get_model_detection_function(detection_model)
# 从 pipeline config 读取 label_map 信息
label_map_path = configs['eval_input_config'].label_map_path
label_map = label_map_util.load_labelmap(label_map_path)
categories = label_map_util.convert_label_map_to_categories(
label_map,
max_num_classes=label_map_util.get_max_label_map_index(label_map),
use_display_name=True)
category_index = label_map_util.create_category_index(categories)
label_map_dict = label_map_util.get_label_map_dict(label_map, use_display_name=True)
# 读图片
image = cv2.imread(PATH_TO_IMAGE)
image_rgb = cv2.cvtColor(image, cv2.COLOR_BGR2RGB)
input_tensor = tf.convert_to_tensor(np.expand_dims(image_rgb, 0), dtype=tf.float32)
# 图片在模型中正向传播
detections, predictions_dict, shapes = detect_fn(input_tensor)
# Draw the results of the detection (aka 'visulaize the results')
# 绘画结果
vis_util.visualize_boxes_and_labels_on_image_array(
image,
detections['detection_boxes'][0].numpy(),
detections['detection_classes'][0].numpy().astype(int),
detections['detection_scores'][0].numpy(),
category_index,
use_normalized_coordinates=True,
max_boxes_to_draw=200,
min_score_thresh=0.6,
agnostic_mode=False)
# All the results have been drawn on image. Now display the image.
cv2.imshow('Object detector', image)
# Press any key to close the image
cv2.waitKey(0)
# Clean up
cv2.destroyAllWindows()
注意修改读取路径
③模型转换.pb 到 .tflite
查看 tensorflow Lite官网文档
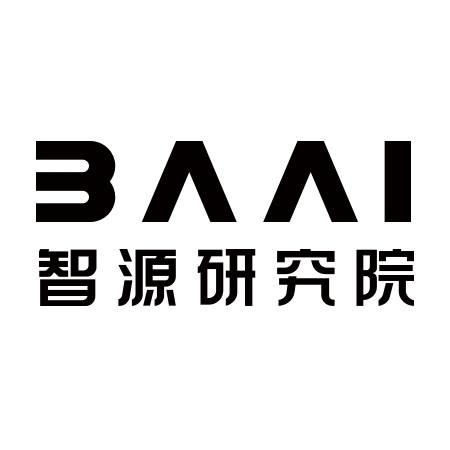
欢迎来到由智源人工智能研究院发起的Triton中文社区,这里是一个汇聚了AI开发者、数据科学家、机器学习爱好者以及业界专家的活力平台。我们致力于成为业内领先的Triton技术交流与应用分享的殿堂,为推动人工智能技术的普及与深化应用贡献力量。
更多推荐
所有评论(0)